How data is transforming the music industry
by Brian Moon
Fifteen years ago, Steve Jobs introduced the iPod. Since then, most music fans have understood this has radically changed how they listen to music.
Less understood are the ways that raw information – accumulated via downloads, apps and online searches – is influencing not only what songs are marketed and sold, but which songs become hits.
Decisions about how to market and sell music, to some extent, still hinge upon subjective assumptions about what sounds good to an executive, or which artists might be easier to market. Increasingly, however, businesses are turning to big data and the analytics that can help turn this information into actions.
Big data is a term that reflects the amount of information people generate – and it’s a lot. Some estimate that today, humans generate more information in one minute than in every moment from the earliest historical record through 2000.
Unsurprisingly, harnessing this data has shaped the music industry in radical new ways.
When it was all about the charts
In the 20th century, decisions about how to market and sell music were based upon assumptions about who would buy it or how they would hear it.
At times, purely subjective assumptions would guide major decisions. Some producers, like Phil Spector and Don Kirshner, earned reputations for their “golden ears” – their ability to intuit what people would want to listen to before they heard it. (If you aren’t aware of the SNL parody of this phenomenon, take a second to see “More Cowbell.”) Eventually, record companies incorporated more market-based objective information through focus groups, along with sheet music and record sales.
But the gold standard of information in the music industry became the “charts,” which track the comparative success of one recording against others.
Music charts have typically combined two pieces of information: what people are listening to (radio, jukeboxes and, today, streaming) and what records they’re buying.
Charts like the Billboard Hot 100 measure the exposure of a recording. If a song is in the first position on a list of pop songs, the presumption is that it’s the most popular – the most-played song on the radio, or the most-purchased in record stores. In the 1920s through the 1950s, when record charts began to appear in Billboard, they were compiled from sales information provided by select shops where records were sold. The number of times a recording played on the radio began to be incorporated into the charts in the 1950s.
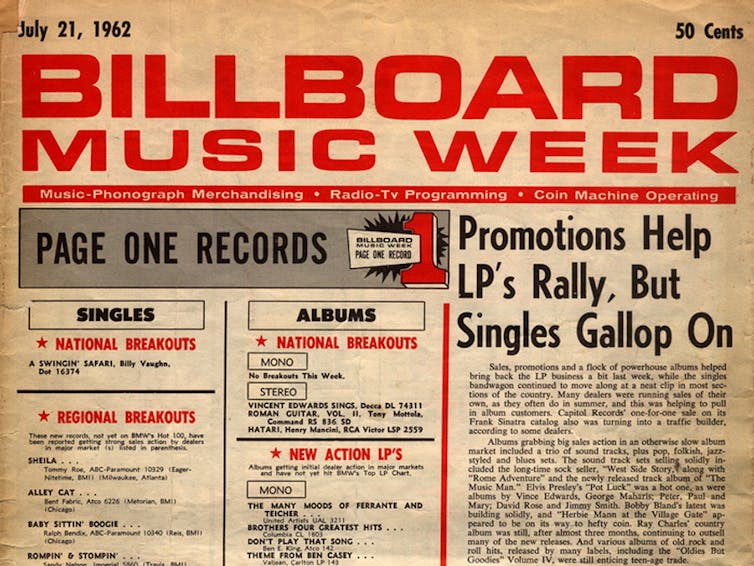
While charts attempt to be objective, they don’t always capture musical tastes and listening habits. For example, in the 1950s, artists started appearing on multiple charts presumed to be distinct. When Chuck Berry made a recording of “Maybellene” that simultaneously appeared in the country and western, rhythm and blues, and pop charts, it upended certain assumptions that undergirded the music industry – specifically, that the marketplace was as segregated as the United States. Simply put, the industry assumed that pop and country were Caucasian, while R&B was African-American. Recordings like “Maybellene” and other “crossover” hits signaled that subjective tastes weren’t being accurately measured.
In the 1990s, chart information incorporated better data, with charts automatically being tracked via scans at record stores. Once sales data began to be accumulated across all stores using Nielsen Soundscan, some larger assumptions about what people were listening to were challenged. The best-selling recordings in the early 1990s were often country and hip-hop records, even though America’s radio stations during the 1980s had tended to privilege classic rock.
Record charts are constantly evolving. Billboard magazine has the longest-running series of charts evaluating different genres and styles of music, and so it makes a good standard for comparison. Yet new technology has made this system a bit problematic. For example, data generated from Pandora weren’t added to the Billboard charts until January of this year.
The end of genre?
Today, companies are trying to make decisions relying on as few assumptions as possible. Whereas in the past, the industry relied primarily on sales and how often a songs were played on the radio, they can now see what specific songs people are listening to, where they are hearing it and how they are consuming it.
On a daily basis, people generate 2.5 exabytes of data, which is the equivalent to 250,000 times all of the books in the Library of Congress. Obviously, not all of this data is useful to the music industry. But analytical software can utilize some of it to help the music industry understand the market.
The Musical Genome, the algorithm behind Pandora, sifts through 450 pieces of information about the sound of a recording. For example, a song might feature the drums as being one of the loudest components of the sound, compared to other features of the recording. That measurement is a piece of data that can be incorporated into the larger model. Pandora uses these data to help listeners find music that is similar in sound to what they have enjoyed in the past.
This approach upends the 20th-century assumptions of genre. For example, a genre such as classic rock can become monolithic and exclusionary. Subjective decisions about what is and isn’t “rock” have historically been sexist and racist.
With Pandora, the sound of a recording becomes much more influential. Genre is only one of 450 pieces of information that’s being used to classify a song, so if it sounds like 75 percent of rock songs, then it likely counts as rock.
Meanwhile, Shazam began as an idea that turned sound into data. The smartphone app takes an acoustic fingerprint of song’s sound to reveal the artist, song title and album title of the recording. When a user holds his phone toward a speaker playing a recording, he quickly learns what he is hearing.
The listening habits of Shazam’s 120 million active users can be viewed in real time, by geographic location. The music industry now can learn how many people, when they heard a particular song, wanted to know the name of the singer and artist. It gives real-time data that can shape decisions about how – and to whom – songs are marketed, using the preferences of the listeners. Derek Thompson, a journalist who has examined data’s affects on the music industry, has suggested that Shazam has shifted the power of deciding hits from the industry to the wisdom of a crowd.
The idea of converting a recording’s sound into data has also led to a different way of interpreting this information.
If we know the “sound” of past hits – the interaction between melody, rhythm, harmony, timbre and lyrics – is it possible to predict what the next big hit will be? Companies like Music Intelligence Solutions, Inc., with its software Uplaya, will compare a new recording to older recordings to predict success. The University of Antwerp in Belgium conducted a study on dance songs to create a model that had a 70 percent likelihood of predicting a hit.
Of course, YouTube might tend to cluster songs by genre in its search algorithm, but it’s increasingly clear that the paradigms that have defined genres are less applicable now than ever before.
What happens next?
Even as new information becomes available, old models still help us organize that information. Billboard Magazine now has a Social 50 chart which tracks the artists most actively mentioned on the world’s leading social media sites.
In a way, social media can be thought of as analogous to the small musical scenes of the 20th century, like New York City’s CBGB or Seattle’s Sub Pop scene. In Facebook groups or on Twitter lists, some dedicated and like-minded fans are talking about the music they enjoy – and record companies want to listen. They’re able to follow how the “next big thing” is being voraciously discussed within a growing and devoted circle of fans.
Streaming music services are increasingly focused upon how social media is intertwined with the listening experience. The Social 50 chart is derived from information gathered by the company Next Big Sound, which is now owned by Pandora. In 2015, Spotify acquired the music analytics firm The Echo Nest, while Apple Music acquired Semetric .
Songwriters and distributors now know – more than ever – how people listen to music and which sounds they seem to prefer.
But did people like OMI’s 2015 hit “Cheerleader” because of its sound and its buzz on social media – as Next Big Sound predicted? Or did it spread on these networks only because it possessed many of the traits of a successful record?
Does taste even matter? You’d like to think you listen to what you enjoy, not what the industry predicts you’ll like based on data. But is your taste your own? Or will the feedback loop – where what you’ve enjoyed in the past shapes what you hear today – change what you’ll like in the future?